Entrepreneurial Geekiness
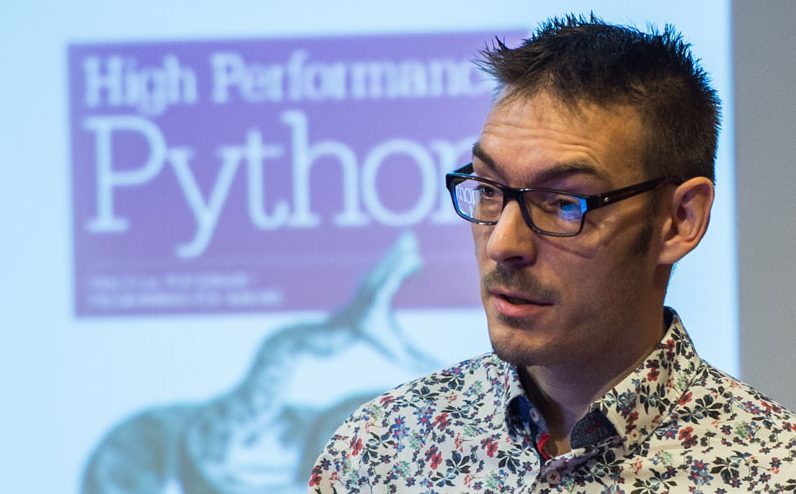
PyConUK 2017, PyDataCardiff and “Machine Learning Libraries You’d Wish You’d Known About”
A week back I had the pleasure to talk on machine learning at PyConUK 2017 in the inaugural PyDataCardiff track. Tim Vivian-Griffiths and colleagues did a wonderful job building our second PyData conference event in the UK. The PyConUK conference just keeps getting better – 700 folk, 5 tracks, a huge kids track and lots of sub-events. Pythontastic! Cat Lamin has a lovely write-up of the main conference.
If you’re interested in PyDataCardiff then note that Tim has setup an announcements-list, join it to hear about meetup events around Cardiff and Bristol.
I spoke on the Saturday on “Machine Learning Libraries You’d Wish You’d Known About” (slides here) – this is a precis of topics that I figured out this year:
- Using Pandas multi-core with Dask
- Automating your machine learning with TPOT on sklearn
- Visualising your machine learning with YellowBrick
- Explaining why you get certain machine learning answers with ELI5 and LIME
- See my “Explaining Regression” Notebook for lots of examples with YellowBrick, ELI5, LIME and more (I used this to build my talk)
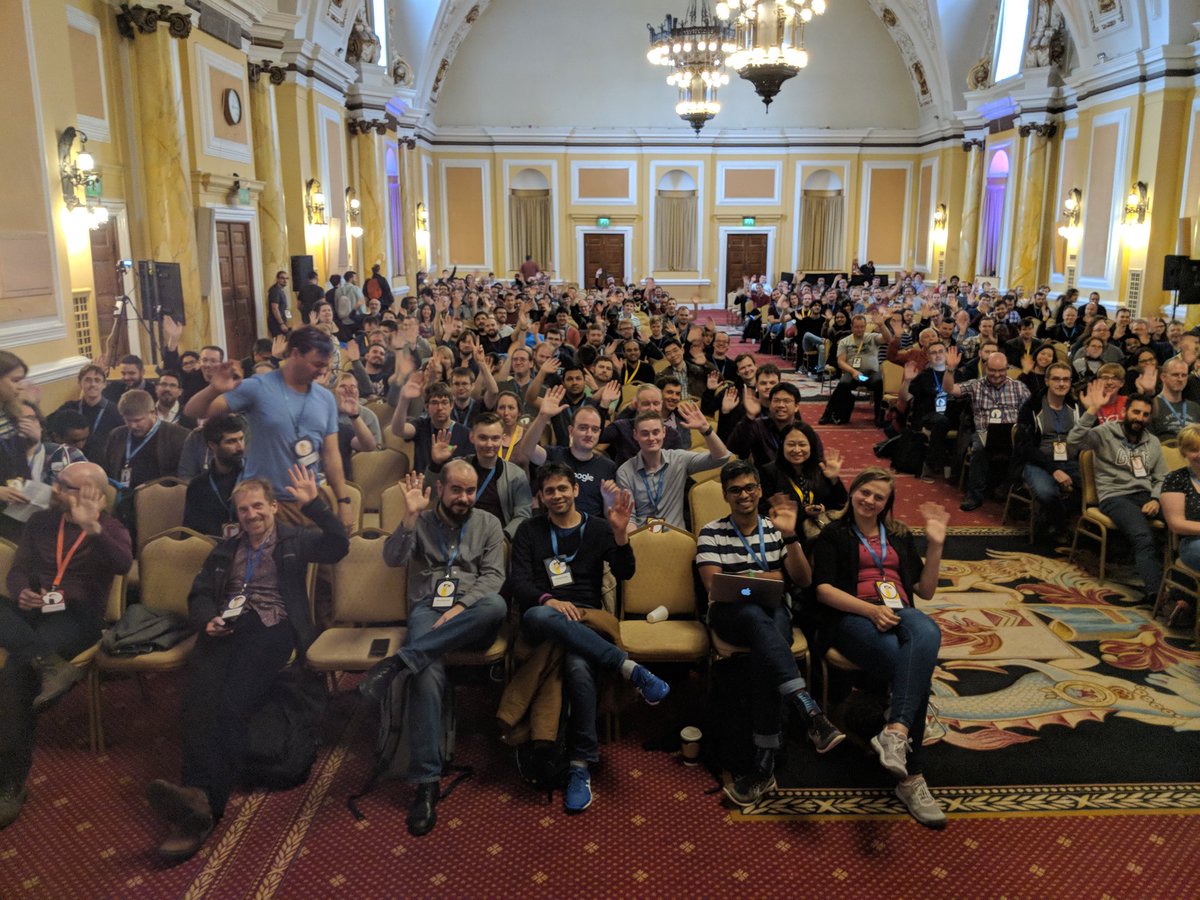
As with last year I was speaking in part to existing engineers who are ML-curious, to show ways of approaching machine learning diagnosis with an engineer’s-mindset. Last year I introduced Random Forests for engineers using a worked example. Below you’ll find for video for this year’s talk:
I’m planning to do more teaching on data science and Python in 2018 – if this might interest you, please join my training mailing list. Posts will go out rarely to announce new public and private training sessions that’ll run in the UK.
At the end of my talk I made a request of the audience, I’m going to start doing this more frequently. My request was “please send me a physical postcard if I taught you something” – I’d love to build up some evidence on my wall that these talks are useful. I received my first postcard a few days back, I’m rather stoked. Thank you Pieter! If you want to send me a postcard, just send me an email. Do please remember to thank your speakers – it is a tiny gesture that really carries weight.
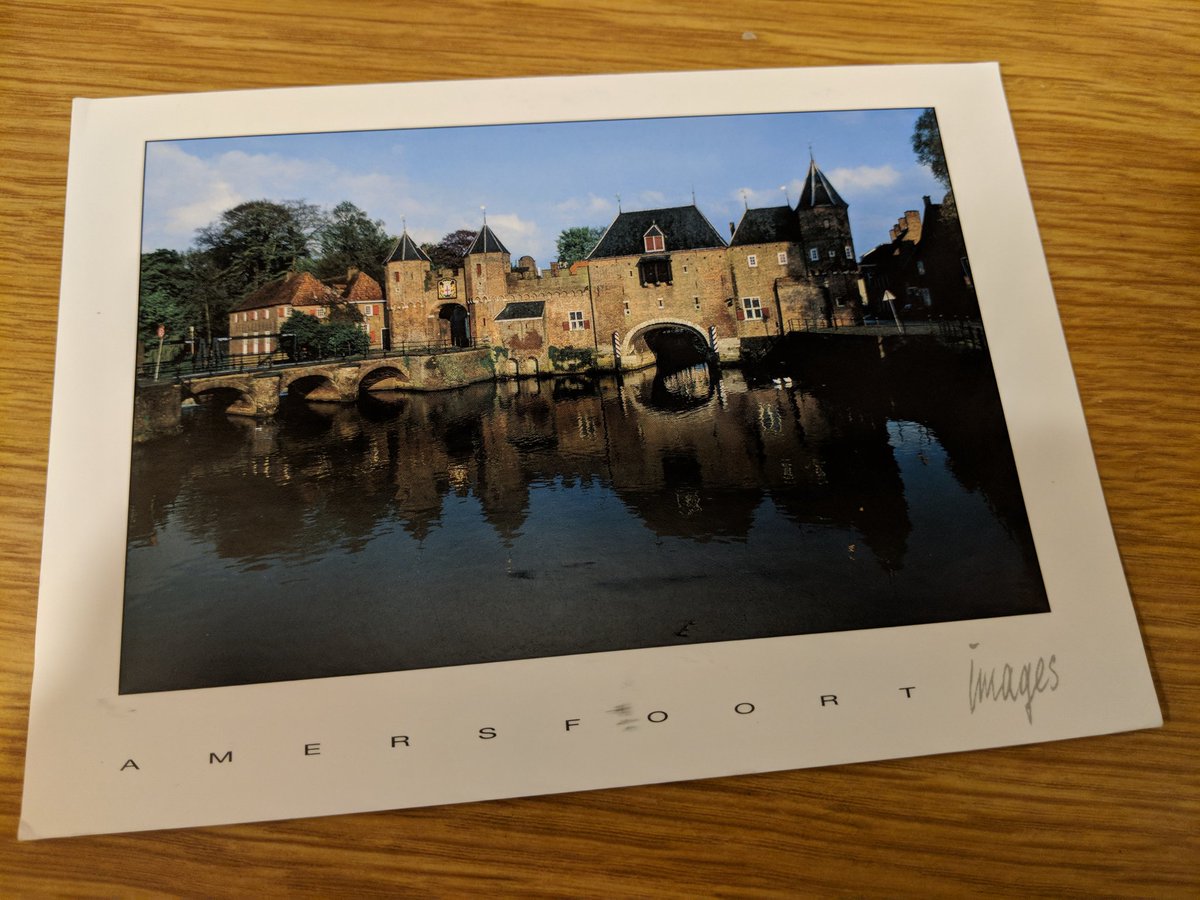
Thanks to O’Reilly I also got to participate in another High Performance Python signing, this time with Steve Holden (Python in a Nutshell: A Desktop Quick Reference), Harry Percival (Test-Driven Development with Python 2e) and Nicholas Tollervy (Programming with MicroPython):
I want to say a huge thanks to everyone I met – I look forward to a bigger and better PyConUK and PyDataCardiff next year!
If you like data science and you’re in the UK, please do check-out our PyDataLondon meetup. If you’re after a job, I have a data scientist’s jobs list.
Ian is a Chief Interim Data Scientist via his Mor Consulting. Sign-up for Data Science tutorials in London and to hear about his data science thoughts and jobs. He lives in London, is walked by his high energy Springer Spaniel and is a consumer of fine coffees.
Kaggle’s Mercedes-Benz Greener Manufacturing
Kaggle are running a regression machine learning competition with Mercedes-Benz right now, it closes in a week and runs for about 6 weeks overall. I’ve managed to squeeze in 5 days to have a play (I managed about 10 days on the previous Quora competition). My goal this time was to focus on new tools that make it faster to get to ‘pretty good’ ML solutions. Specifically I wanted to play with:
- TPOT “auto scikit-learn” (but not the auto-sklearn package which is related)
- The YellowBrick sklearn visualiser
Most of the 5 days were spent either learning the above tools or making some suggestions for YellowBrick, I didn’t get as far as creative feature engineering. Currently I’m in the top 50th percentile Now the competition has finished I’m at rank 1497 (top 37th percentile) on the leaderboard using raw features, some dimensionality reduction and various estimators, with 5 days of effort.
TPOT is rather interesting – it uses a genetic algorithm approach to evolve the hyperparameters of one or more (Stacked) estimators. One interesting outcome is that TPOT was presenting good models that I’d never have used – e.g. an AdaBoostRegressor & LassoLars or GradientBoostingRegressor & ElasticNet.
TPOT works with all sklearn-compatible classifiers including XGBoost (examples) but recently there’s been a bug with n_jobs and multiple processes. Due to this the current version had XGBoost disabled, it looks now like that bug has been fixed. As a result I didn’t get to use XGBoost inside TPOT, I did play with it separately but the stacked estimators from TPOT were superior. Getting up and running with TPOT took all of 30 minutes, after that I’d leave it to run overnight on my laptop. It definitely wants lots of CPU time. It is worth noting that auto-sklearn has a similar n_jobs bug and the issue is known in sklearn.
It does occur to me that almost all of the models developed by TPOT are subsequently discarded (you can get a list of configurations and scores). There’s almost certainly value to be had in building averaged models of combinations of these, I didn’t get to experiment with this.
Having developed several different stacks of estimators my final combination involved averaging these predictions with the trustable-model provided by another Kaggler. The mean of these three pushed me up to 0.55508. My only feature engineering involved various FeatureUnions with the FunctionTransformer based on dimensionality reduction.
YellowBrick was presented at our PyDataLondon 2017 conference (write-up) this year by Rebecca (we also did a book signing). I was able to make some suggestions for improvements on the RegressionPlot and PredictionError along with sharing some notes on visualising tree-based feature importances (along with noting a demo bug in sklearn). Having more visualisation tools can only help, I hope to develop some intuition about model failures from these sorts of diagrams.
Here’s a ResidualPlot with my added inset prediction errors distribution, I think that this should be useful when comparing plots between classifiers to see how they’re failing:
Ian is a Chief Interim Data Scientist via his Mor Consulting. Sign-up for Data Science tutorials in London and to hear about his data science thoughts and jobs. He lives in London, is walked by his high energy Springer Spaniel and is a consumer of fine coffees.
Kaggle’s Quora Question Pairs Competition
Kaggle‘s Quora Question Pairs competition has just closed, I’m pleased to say that with 10 days effort I ranked in the top 39th percentile (rank 1346 of 3396 in the private leaderboard). Having just run and spoken at PyDataLondon 2017, taught ML in Romania and worked on several client projects I only freed up time right at the end of this competition. Despite joining at the end I had immense fun – this was my first ‘proper’ Kaggle competition.
I figured a short retrospective here might be a useful reminder to myself in the future. Things that worked well:
- Use of github, Jupyter Notebooks, my research module template
- Python 3.6, scikit-learn, pandas
- RandomForests (some XGBoost but ultimately just RFs)
- Dask (great for using all cores when feature engineering with Pandas apply)
- Lots of text similarity measures, word2vec, some Part of Speech tagging
- Some light text clean-up (punctuation, whitespace, some mixed case normalisation)
- Spacy for PoS noun extraction, some NLTK
- Splitting feature generation and ML exploitation into different Notebooks
- Lots of visualisation of each distance measure by class (mainly matplotlib histograms on single features)
- Fully reproducible Notebooks with fixed seeds
- Debugging code to diagnose the most-wrong guesses from the model (pulling out features and the raw questions was often enough to get a feel for “what it missed” which lead to thoughts on new features that might help)
Things that I didn’t get around to trying due to lack of time:
- PoS named entities in Spacy, my own entity recogniser
- GloVe, wordrank, fasttext
- Clustering around topics
- Text clean-up (synonyms, weights & measures normalisation)
- Use of external corpus (e.g. Stackoverflow) for TF-IDF counts
- Dask on EC2
Things that didn’t work so well:
- Fully reproducible Notebooks (great!) to generate features with no caching of no-need-to-rebuild-yet-again features, so I did a lot of recalculating features (which really hurt in the last 2 days) – possible solution below with named columns
- Notebooks are still a PITA for debugging, attaching a console with –existing works ok until things start to crash and then it gets sticky
- Running out of 32GB of RAM several times on my laptop and having a semi-broken system whilst trying to persist partial models to disk – I should have started with an AWS deployment earlier so I could easily turn on more cores+RAM as needed
- I barely checked the Kaggle forums (only reading the Notebooks concerning the negative resampling requirement) so I missed a whole pile of tricks shared by others, some I folded in on the last day but there’s a huge pile that I missed – I think I might have snuck into the top 20% of rankings if I’d have used this public information
- Calibrating RandomForests (I’m pretty convinced I did this correctly but it didn’t improve things, I’m not sure why)
Dask definitely made parallelisation easier with only a few lines of overhead in a function beyond a normal call to apply. The caching, if using something like luigi, would add a lot of extra engineered overhead – not so useful in a rapidly iterating 10 day competition.
I think next time I’ll try using version-named columns in my DataFrames. Rather than having e.g. “unigram_distance_raw_sentences” I might add “_v0”, if that calculation process is never updated then I can just use a pre-built version of the column. This is a poor-mans caching strategy. If any dependencies existed then I guess luigi/airflow would be the next step. For now at least I think a version number will solve my most immediate time-sink in recent days.
I hope to enter another competition soon. I’m also hoping to attend the London Kaggle meetup at some point to learn from others.
Ian is a Chief Interim Data Scientist via his Mor Consulting. Sign-up for Data Science tutorials in London and to hear about his data science thoughts and jobs. He lives in London, is walked by his high energy Springer Spaniel and is a consumer of fine coffees.
PyDataLondon 2017 Conference write-up
Several weeks back we ran our 4th PyDataLondon (2017) conference – it was another smashing success! This builds on our previous 3 years of effort (2016, 2015, 2014) building both the conference and our over-subscribed monthly meetup. We’re grateful to our host Bloomberg for providing the lovely staff, venue and catering.
Really got inspired by @genekogan’s great talk on AI & the visual arts at @pydatalondon @annabellerol
Each year we try some new ideas – this year we tried:
- 4 hour Hackathon on NLP to help our keynote speakers from FullFact to try new ideas
- “Making your first open source submission” session to help 30 people step closer to contributing to open source (thanks Katy and Nick for leading that session!)
- A scavenger hunt
- A crazy pub-quiz (James Powell is responsible for blowing quite a few minds) on Saturday evening
- A longer-running Slack channel
- New co-chair Ruby and review committee lead Linda
- More financial support for speakers and attendees to increase diversity
- Several unconference slots (filled by volunteers on the day) spoke on Bayesian statistics and other topics
- Our first Women @ PyData reception just before the conference started
- More book signing
- Fast video turnaround into YouTube
- A drone giveaway (by sponsor [and my business partner] Endava)
pros: Great selection of talks for all levels and pub quiz cons: on a weekend, pub quiz (was hard). Overall would recommend 9/10 @harpal_sahota
We’re very thankful to all our sponsors for their financial support and to all our speakers for donating their time to share their knowledge. Personally I say a big thank-you to Ruby (co-chair) and Linda (review committee lead) – I resigned both of these roles this year after 3 years and I’m very happy to have been replaced so effectively (ahem – Linda – you really have shown how much better the review committee could be run!). Ruby joined Emlyn as co-chair for the conference, I took a back-seat on both roles and supported where I could. Our volunteer team great again – thanks Agata for pulling this together.
I believe we had 20% female attendees – up from 15% or so last year. Here’s a write-up from Srjdan and another from FullFact (and one from Vincent as chair at PyDataAmsterdam earlier this year) – thanks!
#PyDataLdn thank you for organising a great conference. My first one & hope to attend more. Will recommend it to my fellow humanists! @1208DL
For this year I’ve been collaborating with two colleagues – Dr Gusztav Belteki and Giles Weaver – to automate the analysis of baby ventilator data with the NHS. I was very happy to have the 3 of us present to speak on our progress, we’ve been using RandomForests to segment time-series breath data to (mostly) correctly identify the start of baby breaths on 100Hz single-channel air-flow data. This is the precursor step to starting our automated summarisation of a baby’s breathing quality.
Slides here and video below:
This updates our talk at the January PyDataLondon meetup. This collaboration came about after I heard of Dr. Belteki’s talk at PyConUK last year, whilst I was there to introduce RandomForests to Python engineers. You’re most welcome to come and join our monthly meetup if you’d like.
Many thanks to all of our sponsors again including Bloomberg for the excellent hosting and Continuum for backing the series from the start and NumFOCUS for bringing things together behind the scenes (and for supporting lots of open source projects – that’s where the money we raise goes to!).
There are plenty of other PyData and related conferences and meetups listed on the PyData website – if you’re interested in data then you really should get along. If you don’t yet contribute back to open source (and really – you should!) then do consider getting involved as a local volunteer. These events only work because of the volunteered effort of the core organising committees and extra hands (especially new members to the community) are very welcome indeed.
I’ll also note – if you’re in London or the south-east of the UK and you want to get a job in data science you should join my data scientist jobs email list, a set of companies who attended the conference have added their jobs for the next posting. Around 600 people are on this list and around 7 jobs are posted out every 2 weeks. Your email is always kept private.
Ian is a Chief Interim Data Scientist via his Mor Consulting. Sign-up for Data Science tutorials in London and to hear about his data science thoughts and jobs. He lives in London, is walked by his high energy Springer Spaniel and is a consumer of fine coffees.
Introduction to Random Forests for Machine Learning at the London Python Meetup
Last night I had the pleasure of returning to London Python to introduce Random Forests (this builds on my PyConUK 2016 talk from September). My goal was to give a pragmatic introduction to solving a binary classification problem (Kaggle’s Titanic) using scikit-learn. The talk (slides here) covers:
- Organising your data with Pandas
- Exploratory Data Visualisation with Seaborn
- Creating a train/test set and using a Dummy Classifier
- Adding a Random Forest
- Moving towards Cross Validation for higher trust
- Ways to debug the model (from the point of view of a non-ML engineer)
- Deployment
- Code for the talk is a rendered Notebook on github
I finished with a slide on Community (are you contributing? do you fulfill your part of the social contract to give back when you consume from the ecosystem?) and another pitching PyDataLondon 2017 (May 5-7th). My colleague Vincent is over from Amsterdam – he pitched PyDataAmsterdam (April 8-9th). The Call for Proposals is open for both, get your talk ideas in quickly please.
I’m really happy to see the continued growth of the London Python meetup, this was one of the earliest meetups I ever spoke at. The organisers are looking for speakers – do get in touch with them via meetup to tell them what you’d like to talk on.
Ian is a Chief Interim Data Scientist via his Mor Consulting. Sign-up for Data Science tutorials in London and to hear about his data science thoughts and jobs. He lives in London, is walked by his high energy Springer Spaniel and is a consumer of fine coffees.
Read my book
Oreilly High Performance Python by Micha Gorelick & Ian Ozsvald AI Consulting
Co-organiser
Trending Now
1Leadership discussion session at PyDataLondon 2024Data science, pydata, RebelAI2What I’ve been up to since 2022pydata, Python3Upcoming discussion calls for Team Structure and Buidling a Backlog for data science leadsData science, pydata, Python4My first commit to PandasPython5Skinny Pandas Riding on a Rocket at PyDataGlobal 2020Data science, pydata, PythonTags
Aim Api Artificial Intelligence Blog Brighton Conferences Cookbook Demo Ebook Email Emily Face Detection Few Days Google High Performance Iphone Kyran Laptop Linux London Lt Map Natural Language Processing Nbsp Nltk Numpy Optical Character Recognition Pycon Python Python Mailing Python Tutorial Robots Running Santiago Seb Skiff Slides Startups Tweet Tweets Twitter Ubuntu Ups Vimeo Wikipedia