Entrepreneurial Geekiness
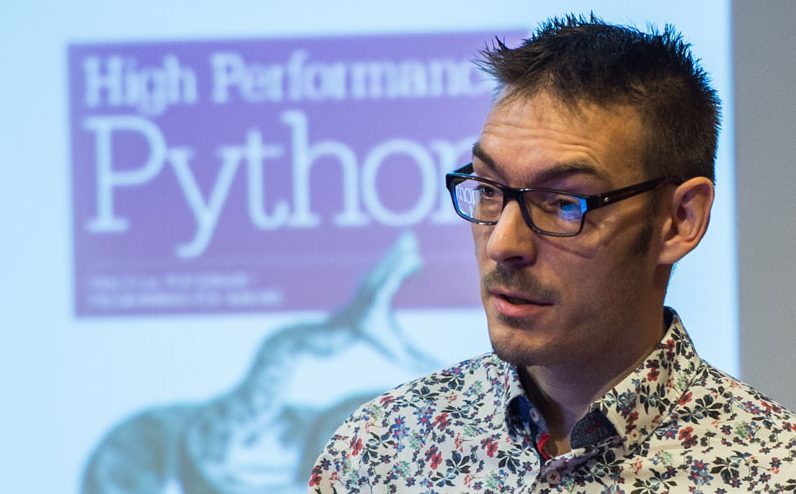
Statistically Solving Sneezes and Sniffles – a Work in Progress Report at PyDataLondon 2016
This is a Work in Progress report, presented this morning at my PyDataLondon 2016 conference. A group of 4 of us are modelling a year’s worth of self-reported data from my wife around her allergies – we’re learning to model which environmental conditions cause her sneezes such that she might have more control over her antihistamine use. Join the email updates list for low-volume updates about this project.
I really should have warned my audience that I was about to photograph them (honest – they seemed to enjoy the talk!):
Emily created the Allergy Tracker (open src) iPhone app a year ago, she logs every sneeze, antihistamine, alcoholic drink, runny nose and more. She’s sneezed for 20 years and by heck, we wondered if we could apply some Data Science to the problem to see if her symptoms correlate with weather, food and pollution. I’m pleased to say we’ve made some progress – it looks like humidity is connected to her propensity to use an antihistamine.
This talk (co-presented with Giles Weaver) discusses the data, the app, our approach to analysis and our tools (including Jupyter, scikit-learn, R, Anaconda and Seaborn) to build a variety of machine learned models to try to model antihistamine usage against external factors. Here are the slides:
Now we’re moving forward to a couple of other participants (we’d like a few more to join us – if you’re on iOS and in London and can commit to 3 months consistent usage we’ll try to tell you what drives your sneezes). We also have academic introductions so we can validate our ideas (and/or kick them into the ground and try again!).
This is the second full day of the conference – we have 330 attendees and we’ve had 2 great keynote speakers and a host of wonderful talks and tutorials (yesterday). Tonight we have our conference party. I’m super happy with how things are progressing – many thanks to all of our speakers, volunteers, Bloomberg and our sponsors for making this work so well.
Update – featured in Mode Analytics #23.
Update – I did a follow-up talk at ODSC 2016 with notes on a new medication that we’ve tried.
Ian is a Chief Interim Data Scientist via his Mor Consulting. Sign-up for Data Science tutorials in London and to hear about his data science thoughts and jobs. He lives in London, is walked by his high energy Springer Spaniel and is a consumer of fine coffees.
Will we see “[module] on Python 3.4+ is free but only paid-support for Python 2.7”?
I’m curious about the transition in our ecosystem from Python 2 to Python 3. On stage at our monthly PyDataLondon meetups I’m known to badger folk to take the step and upgrade to reduce the support burden on developers. The transition gathers pace but it still feels slow. I’ve noted my recommendations for moving to Python 3+ at the end. See also the reddit discussion.
I’m wondering – when will we see the point where open source projects say “We support Python 3.x for free but if you want bugs fixed for Python 2.7, you’ll have to pay“? I’m not saying “if”, but “when”. There’s already one example below and others will presumably follow.
In the last couple of years a slew of larger projects have dropped or are dropping support for Python 2.6 – numpy (some discussion), pandas, scipy, matplotlib, NLTK, astropy, dask, ipython, django, numba, twisted, scrapy. Good – Python 2.6 was deprecated when 2.7 was released in 2010 (that’s 6 years ago!).
The position of the matplotlib and django teams is clearly “Python 2.7 and Python 3.4+”. Django states that Python 2.7 will be supported until the 2020 sunset date:
“As a final heads up, Django 1.11 is likely to be the last version to support Python 2.7 as it will be supported until the end of Python 2 upstream support in 2020. We’ve adopted a Python version support policy…”
We can expect the larger projects to support legacy userbases with a mix of Python 2.7 and 3.4+ for 3.5 years (at least until 2020). After this we should expect projects to start to drop 2.7 support, some (hopefully) more aggressively than others.
What about smaller projects? Several have outright dropped Python 2.7 support already – errbot (2016 is the last Python 2.7-supported year), nikola, python-thumbnails – or never supported it – wordfreq, featherweight. Which others have I missed? Update – JupyterHub (cheers Thomas) too. IPython 6.0 in 2007 will be Python 3.4+ only (IPython 5.0 is the last supported Python 2.7 release). mitmproxy has just switched to a Python-3-only branch (Sept 2016).
More interestingly David MacIver (of Hypothesis) stated a while back that he’d support Python 2.7 for free but Python 2.6 would be a paid support option. He’s also tagged (regardless of version) a bunch of bugs that can be fixed for a fee. Viewflow is another – Python 3.4 is free for non-commercial use but a commercial license or support for Python 2.7 requires a fee. Asking for money to support old, PITA or difficult options seems mightily sensible. I guess we’ll see this first for tools that have a good industrial userbase who’d be used to paying for support (like Viewflow).
Aaron Meurer (lead dev on SymPy) has taken the position that library leaders should pledge for a switch to Python 3.x only by 2020. The pledge shows that scikit-bio is about to go Python 3-only and that IPython 6.x+ will be Python 3 only (from 2017). Increasingly we’ll see new libraries adding the shiny features for their Python 3 branch only.
What next? I imagine most new smaller projects will be Python 3.4+ (probably 3.5+ only soon), they’ll have no legacy userbase to support. They could widen their potential userbase by supporting Python 2.7 but this window only exists for 3 years and those users will have to upgrade anyhow. So why bother going backwards?
Once users notice that cooler new toys are Python 3.4+ only they’ll want to upgrade (e.g. NetworKit is Python 3.3+ only for high volume graph network analysis). They’ll only hold back if they’re supporting legacy internal systems (which will be the case for an awful lot of people). We’ll see this more as we get closer to 2020. What about after 2020?
I guess many companies will be slow to jump to Python 3 (it’d take a lot of effort for no practical improvement), so I’d imagine separate groups will start to support Python 2.7 libraries as forks. Hopefully the main library developers will drop support fairly quickly, to stop open source (cost-free) developers having a tax on their time supporting both platforms.
Separate evidence – Drupal 6 adopted a commercial-support-for-old-versions policy (thanks @chx). It is also worth noting that Ubuntu 16.04 LTS ships without Python 2. Microsoft and Brett Cannon have discussed the benefits of moving to Python 3+ recently.
My recommendations (coming from a Senior Industrial Data Scientist with 15+ years commercial experience and 10+ years using Python):
- Where feasible – all new projects must use Python 3.5+ (e.g. Proof of Concepts, Research, new isolated systems) – this is surprisingly easy
- If Python 2.7 compatibility is required – write all new code in a Python 3.5+ compatible way (1, 2, 3, 4), make extensive tests for the later inevitable migration (you already have good test-coverage, right?)
- Accept that support for Python 2.7 gets turned off in 3.5 years and that all Python 2.7 code written now will likely have to be migrated later (this is a business cost that you can estimate now)
- Accept that as we get closer to 2020 more programmers (both new and experienced) will be using Python 3.5+, so support for Python 2.7-based libraries will inevitably decline (there’s a big business risk here)
Graham and I did a lightning talk on jumping to Python 3 six months back, there’s a lot of new features in Python 3.4+ that will make your life easier (and make your code safer, so you burn less time hunting for problems). Jake also discussed the general problem for scientists back in 2013, it’ll be lovely when we get past this (now-very-boring) discussion.
Ian is a Chief Interim Data Scientist via his Mor Consulting. Sign-up for Data Science tutorials in London and to hear about his data science thoughts and jobs. He lives in London, is walked by his high energy Springer Spaniel and is a consumer of fine coffees.
Convert London Oyster (Travel) PDFs to Pandas DataFrames
As a part of analysing Emily’s allergic rhinitis we want to test whether using the London Underground (notoriously dirty!) increases the likelihood of sneezing. The “black snot” phenomenon is well known to Londoners, possibly the particulates (from oil and metal) cause irritation. You can get updates via our allergic rhinitis analysis mailing list (very very low volume).
Transport for London lets us download a log of journeys – either as a CSV file (just dates and costs, no details) or a PDF file (containing full details of the journey and time). It would be much nicer if they made the data available in a cleanly-formatted open format (e.g. at least a CSV, preferably as HDF5).
The goal is to take the detail-rich PDFs and to build a DataFrame like:
from is_train to
date
2016-01-30 Bus Journey, Route 46 False
2016-01-28 Kentish Town True Leicester Square
2016-01-28 Old Street True Kentish Town
2016-01-28 Leicester Square True Old Street
2016-01-27 Angel True Kentish Town
Using textract (see these Python 3.4 install notes, I also use pdftotext) and a very hacky parser (written this evening, it really is a stateful-messy-hack <sorry>) I can parse a single PDF or a folder to build a Pandas DataFrame of journeys. You’ll find London Oyster PDF to DataFrame Parser here. The output is an HDF5 which can be loaded by Python into Pandas (or R or Matlab or whatever).
Ian is a Chief Interim Data Scientist via his Mor Consulting. Sign-up for Data Science tutorials in London and to hear about his data science thoughts and jobs. He lives in London, is walked by his high energy Springer Spaniel and is a consumer of fine coffees.
PyDataLondon 2016 Call for Proposals Open
Our Call for Proposals for PyDataLondon 2016 (May 6-8) is open until approx. end of February (5ish weeks), you need to get your submission in soon!
If you want to sponsor to talk with 330 cutting edge data scientists – you’d better hurry, we’ve already started signing deals.
In the CfP we’re looking for:
- Stories about successful data science projects (including the highs and lows)
- Machine learning (including Deep Learning) – especially why you used certain algorithms and how you diagnosed features
- Visualisation – have you explained or explored something that’s good to share?
- Data cleaning
- Data process (getting data, understanding it, building models, deploying solutions)
- Industrial and Academic stories
- Big data including Spark
You might also be interested in PyDataAmsterdam on March 12-13th (their Call for Proposals is already open).
We’ve also got a new (temporary URL) webpage for our regular meetups here, this has notes on how to submit a talk to the meetup (not the conference, just the PyDataLondon meetup). Please take a look if you’d like to speak to 200 folk at our monthly meetup.
Ian is a Chief Interim Data Scientist via his Mor Consulting. Sign-up for Data Science tutorials in London and to hear about his data science thoughts and jobs. He lives in London, is walked by his high energy Springer Spaniel and is a consumer of fine coffees.
Data Scientist Jobs in London
Back in January 2015 I announced my Data Science Jobs UK email list. This has grown nicely, several hundred data scientists have joined it and are interested in (mostly) Python related jobs around London with an even split between contract and permanent roles. If you sign-up to the mailing list you’ll get:
- 1-2 plain-ASCII mails a month with a summary of current jobs (typically 4-6), mostly focused around London
- Sometimes the jobs are remote
- Mostly they’re for Python but Matlab and R also come up
I manage the list, your email is never shared and the list is run by mailchimp so you can easily unsubscribe. Active data scientists who attend PyDataLondon can post for free, others can post at a commercial rate (e.g. recruiters and folk in companies). I vet all the jobs to ensure they’re relevant. Drop me an email if you’ve got a relevant job to share.
“After placing a contract ad on this list I was contacted by a number of high quality and enthusiastic data scientists, who all proposed innovative and exciting solutions to my research problem, and were able to explain their proposals clearly to a non-specialist; the quality of responses was so high that I was presented with a real dilemma in choosing who to work with”. – Hazel Wilkinson, Cambridge University
I put the list together to help local data scientists find more relevant jobs, feel free to dip in and out when it might be useful.
Ian is a Chief Interim Data Scientist via his Mor Consulting. Sign-up for Data Science tutorials in London and to hear about his data science thoughts and jobs. He lives in London, is walked by his high energy Springer Spaniel and is a consumer of fine coffees.
Read my book
Oreilly High Performance Python by Micha Gorelick & Ian Ozsvald AI Consulting
Co-organiser
Trending Now
1Leadership discussion session at PyDataLondon 2024Data science, pydata, RebelAI2What I’ve been up to since 2022pydata, Python3Upcoming discussion calls for Team Structure and Buidling a Backlog for data science leadsData science, pydata, Python4My first commit to PandasPython5Skinny Pandas Riding on a Rocket at PyDataGlobal 2020Data science, pydata, PythonTags
Aim Api Artificial Intelligence Blog Brighton Conferences Cookbook Demo Ebook Email Emily Face Detection Few Days Google High Performance Iphone Kyran Laptop Linux London Lt Map Natural Language Processing Nbsp Nltk Numpy Optical Character Recognition Pycon Python Python Mailing Python Tutorial Robots Running Santiago Seb Skiff Slides Startups Tweet Tweets Twitter Ubuntu Ups Vimeo Wikipedia