Entrepreneurial Geekiness
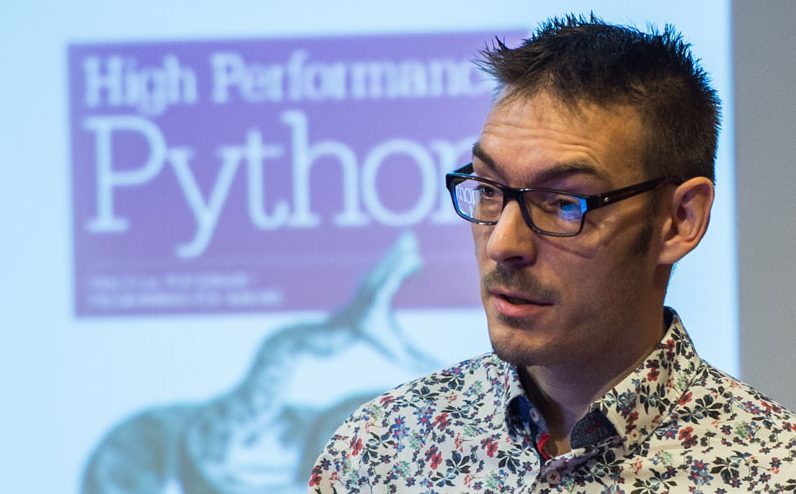
Upcoming discussion calls for Team Structure and Buidling a Backlog for data science leads
I ran another Executives at PyData discussion session for 50+ leaders at our PyDataLondon conference a couple of weeks back. We had great conversation which dug into a lot of topics. I’ve written up notes on my NotANumber newsletter. If you’re a leader of DS and Data Eng teams, you probably want to review those notes.
To follow on the conversations I’m going to run the following two (free) Zoom based discussion sessions. I’ll be recording the calls and adding notes to future newsletters. If you’d like to join, fill in this invite form and I can add you to the calendar invite. You can lurk and listen or – better – join in with questions.
Monday July 11, 4pm (UK time), Data Science Team Structure – getting a good structure for your org, hybrid vs fully remote practices, processes that support your team, how to avoid being left out- Monday August 8th, 4pm (UK time), Backlog & Derisking & Estimation – how to build a backlog, derisking quickly and estimating the value behind your project
I’m expecting a healthy list of issues and good feedback and discussion for both calls. I’ll be sharing an agenda in advance to those who have contacted me. My goal is to turn these into bigger events in the future.
Ian is a Chief Interim Data Scientist via his Mor Consulting. Sign-up for Data Science tutorials in London and to hear about his data science thoughts and jobs. He lives in London, is walked by his high energy Springer Spaniel and is a consumer of fine coffees.
My first commit to Pandas
I’ve used the Pandas data science toolkit for over a decade and I’ve filed a couple of issues, but I’ve never contributed to the source. At the weekend I got to balance the books a little by making my first commit. With this pull request I fixed the recent request to update the pct_change docs to make the final example more readable.
The change was trivial – adding “periods=-1” to the argument and updating the docstring. The build process was a lot more involved – thankfully I was on a call with PyLadies London to try to help others make their first contribution to Pandas and I had organiser Marco Gorelli (a core contributor) to help when needed.
Ultimately it boiled down to setting up a docker environment, running a new example in my shell, updating the relevant docstring on the local filesystem and then following the “contributing to the documentation” guide. My initial commit fell foul of the docstring style rules and the automated checking tools in the docker environment point this out. Once the local filesystem checker scripts were happy I pushed to my fork, created a PR and shortly after everything was done.
All in it took 45 minutes to get the environment setup, another 45 minutes to make my changes and figure out how to run the right scripts, then a bit longer to push and submit a PR (followed by overnight patience before it got picked up by the team).
When I teach my classes I always recommend that a good way to learn new development practices (like the automated use of black & flake8 in a precommit process) is to submit small fixes to open source projects – you learn so much along the way. I’ve not used docker in years and I don’t use automated docstring checking tools, so both presented nice little points for learning. I also have never used the pct_change function in Pandas…and now I have.
If you’ve not yet made a commit to an open source project, do have a think about it – you’ll get lots of hand holding (just be patient, positive and friendly when you leave comments) and you can stick a reference to the result on your CV for bragging rights. And you’ll have made the world a slightly better place.
Ian is a Chief Interim Data Scientist via his Mor Consulting. Sign-up for Data Science tutorials in London and to hear about his data science thoughts and jobs. He lives in London, is walked by his high energy Springer Spaniel and is a consumer of fine coffees.
Skinny Pandas Riding on a Rocket at PyDataGlobal 2020
On November 11th we saw the most ambitious ever PyData conference – PyData Global 2020 was a combination of world-wide PyData groups putting on a huge event to both build our international community and to leverage the on-line only conferences that we need to run during Covid 19.
The conference brought together almost 2,000 attendees from 65 countries with 165 speakers over 5 days on a 5-track schedule. All speaker videos had to be uploaded in advance so they could be checked and then provided ahead-of-time to attendees. You can see the full program here, the topic list was very solid since the selection committee had the best of the international community uploading their proposals.
The volunteer organising committee felt that giving attendees a chance to watch all the speakers at their leisure took away constraints of time zones – but we wanted to avoid the common end result of “watching a webinar” that has plagued many other conferences this year. Our solution included timed (and repeated) “watch parties” so you could gather to watch the video simultaneously with others, and then share discussion in chat rooms. The volunteer organising committee also worked hard to build a “virtual 2D world” with Gather.town – you walk around a virtual conference space (including the speakers’ rooms, an expo hall, parks, a bar, a helpdesk and more). Volunteer Jesper Dramsch made a very cool virtual tour of “how you can attend PyData Global” which has a great demo of how Gather works – it is worth a quick watch. Other conferences should take note.
Through Gather you could “attend” the keynote and speaker rooms during a watch-party and actually see other attendees around you, you could talk to them and you could watch the video being played. You genuinely got a sense that you were attending an event with others, that’s the first time I’ve really felt that in 2020 and I’ve presented at 7 events this year prior to PyDataGlobal (and frankly some of those other events felt pretty lonely – presenting to a blank screen and getting no feedback…that’s not very fulfilling!).
I spoke on “Skinny Pandas Riding on a Rocket” – a culmination of ideas covered in earlier talks with a focus on getting more into Pandas so you don’t have to learn new technologies and see Vaex, Dask and SQLite in action if you do need to scale up your Pythonic data science.
I also organised another “Executives at PyData” session aimed at getting decision makers and team leaders into a (virtual) room for an hour to discuss pressing issues. Given 6 iterations of my “Successful Data Science Projects” training course in London over the last 1.5 years I know of many issues that repeatedly come up that plague decision makers on data science teams. We got to cover a set of issues and talk on solutions that are known to work. I have a fuller write-up to follow.
The conference also enabled a “pay what you can” model for those attending outside of a corporate ticket, this brought in a much wider audience that could normally attend a PyData conference. The goal of the non-profit NumFOCUS (who back the PyData global events) is to fund open source so the goal is always to raise more money and to provide a high quality educational and networking experience. For this on-line global event we figured it made sense to open out the community to even more folk – the “pay what you can” model is regarded as a success (this is the first time we’ve done it!) and has given us some interesting attendee insights to think on.
There are definitely some lessons to learn, notably the on-boarding process was complex (3 systems had to be activated) – the volunteer crew wrote very clear instructions but nonetheless it was a more involved process than we wanted. This will be improved in the future.
I extend my thanks to the wider volunteer organising committee and to NumFOCUS for making this happen!
Ian is a Chief Interim Data Scientist via his Mor Consulting. Sign-up for Data Science tutorials in London and to hear about his data science thoughts and jobs. He lives in London, is walked by his high energy Springer Spaniel and is a consumer of fine coffees.
“Making Pandas Fly” at EuroPython 2020
I’ve had a chance to return to talking about High Performance Python at EuroPython 2020 after my first tutorial on this topic back in 2011 in Florence. Today I spoke on Making Pandas Fly with a focus on making Pandas run faster. This covered:
- Categories and RAM-saving datatypes to make 100-500x speed-ups (well, some of the time) including dtype_diet
- Dropping to NumPy to make things potentially 10x faster (thanks James Powell and his callgraph code)
- Numba for compilation (another 10x!)
- Dask for parallelisation (2-8x!)
- and taking a view on Modin & Vaex
We might ask “why do this” and my answer is “let’s go faster using the tools we already know how to use”. Specifically – without investing time learning a new tool (e.g. Intel SDC, Vaex, Modin, Dask, Spark and more) we can extend our ability to work with larger datasets without leaving the comfort of Pandas so you can get to your answers quicker. This message went down well:
If you’re curious about this and want to go further you might want to look at my upcoming training courses (this includes Higher Performance, Software Engineering and Successful Data Science Projects). If you want tips and you want to stay on top of what I’m working on they join my twice-a-month mailing list (see the link for a recent example post).
Ian is a Chief Interim Data Scientist via his Mor Consulting. Sign-up for Data Science tutorials in London and to hear about his data science thoughts and jobs. He lives in London, is walked by his high energy Springer Spaniel and is a consumer of fine coffees.
Weekish notes
I’ve recently switched back from Sourdough yeast to dried packet yeast mix, given a recipe by a colleague (thanks Nick!). I immediately set to work modifying his recipe (well, cutting out steps if we’re honest). The first loaf looked fine but was bland – I cut out too much salt. The next was really very good (“shop quality”). For the third I used off-boil water for my autolyse and I think the water was still too hot and killed some of the yeast later giving me this dense lump. Later that evening after 2.5 hours I had a luke-warm water repeat loaf and it was brilliant. I confirmed this with toast & jam this morning.
I’ve got quite a log of notes for my two main recipes now and will have a Sourdough on the go again this weekend.
Working with my “still secret” client in a safe haven locked down remote instance I lack most of my usual tools (part by design, part my ignorance during configuration). I’ve got Vi so I’m getting my hands dirty with the underlying operations (hey! :bnext and :e work fine! Ctrl P does some sort of autocomplete! :ls lists my buffers!). This is a little painful and Apache Guacamole’s remote viewer can be troublesome (stripping £ symbols, giving me 3 different keyboard configs depending on when I login, forgetting some of my windows!) but on the whole the setup is working well.
I’ve also had to get down and dirty with Git – no GitK or other fun tools. I’ve discovered some nice light git configs like “git logline” which help with terminal based navigation in our small team.
Training classes are now listed for:
- Software Engineering for Data Scientists (September) – write strong, tested, reliable and defensible code from Notebooks to modules to improve collaboration and resilience
- Higher Performance Python (October) – profile CPU & memory usage, speed up your code, compile where useful and improve your Pandas & Dask to enable faster iteration and faster processing on your projects with minimal effort on your part
- Successful Data Science Projects (November) – discover new process & tools to design data science projects that’ll run successfully, improve collaboration between your team and the wider business (this is built out of 15 years of painful lessons so you don’t have to make the same mistakes!)
Ian is a Chief Interim Data Scientist via his Mor Consulting. Sign-up for Data Science tutorials in London and to hear about his data science thoughts and jobs. He lives in London, is walked by his high energy Springer Spaniel and is a consumer of fine coffees.
Read my book
Oreilly High Performance Python by Micha Gorelick & Ian Ozsvald AI Consulting
Co-organiser
Trending Now
1Upcoming discussion calls for Team Structure and Buidling a Backlog for data science leadsData science, pydata, Python2My first commit to PandasPython3Skinny Pandas Riding on a Rocket at PyDataGlobal 2020Data science, pydata, Python4“Making Pandas Fly” at EuroPython 2020High Performance Python Book, pydata, Python5Weekish notesData science, Python, Week notesTags
Aim Api Artificial Intelligence Blog Brighton Conferences Cookbook Demo Ebook Email Emily Face Detection Few Days Google High Performance Iphone Kyran Laptop Linux London Lt Map Natural Language Processing Nbsp Nltk Numpy Optical Character Recognition Pycon Python Python Mailing Python Tutorial Robots Running Santiago Seb Skiff Slides Startups Tweet Tweets Twitter Ubuntu Ups Vimeo Wikipedia