Entrepreneurial Geekiness
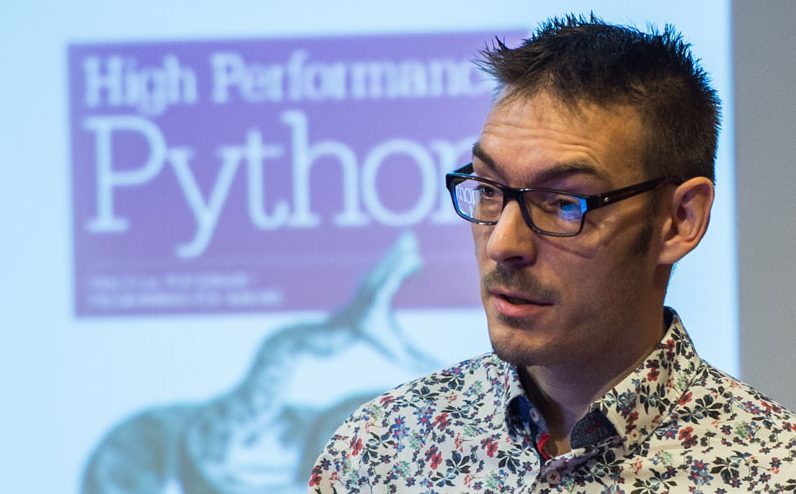
Making Python math 196* faster with shedskin
Dr. Michael Thomas approached me with an interesting A.I. job to see if we could speed up his neural network code from a 10 year old research platform called PlaNet. Using new Sun boxes they weren’t getting the speed-ups they expected, old libs or other monkey business were suspected.
As a first investigation I took Neil Schemenauer’s bpnn.py (a 200 line back-prop artificial neural network library with doc and comparison). The intention was to see how much faster the code might run using psyco and shedskin.
The results were really quite surprising, notes and src follow.
Addition – Leonardo Maffi has written a companion piece showing that his ShedSkin output is 1.5 to 7* slower than hand-coded C. He also shows solutions using the D language and runtimes for Python 2.6 (I use Python 2.5 below). He notes:
“I have translated the Python code to D (using my D libraries) in just few minutes, something like 15-20 minutes, and the translation was mostly painless and sometimes almost mechanical. I have translated the D code to C in many hours. Translating Python => C may require something like 20-30 times the time you need to translate Python => D + my libs. And this despite I have used a rigorous enough method to perform the translation, and despite at the end I am not sure the C code is bug-free. This is an enormous difference.”
End addition.
Addition – Robert Bradshaw has created a Cython version with src, see comments. End addition.
The run-time in minutes for the my harder test case are below. Note that these are averages of 4 runs each:
- Vanilla Python 153 minutes
- Python + Psyco 1.6.0.final.0 57 minutes (2.6* faster)
- Shedskin 0.0.29 0.78 minutes [47 seconds] (196* faster)
The test machines uses Python 2.5.2 on Ubuntu 8.04. The box is an Intel Core Duo 2.4GHz running a single process.
The ‘hard’ problem trains the ANN using 508 patterns with 57 input neurons, 50 hidden and 62 output neurons over 1000 iterations. If you know ANNs then the configuration (0.1 learning rate, 0 momentum) might seem unusual, be assured that this is correct for my researcher’s problem.
There is a shorter version of this problem using just 2 patterns, this is useful if you want to replicate these results but don’t want to wait 3 hours on your first run.
My run times for the shorter problem are (again averaged using 4 runs):
- Vanilla Python 42 seconds
- Python + Psyco 14 seconds
- Shedskin 0.2 seconds (210* faster)
Shedskin has an issue with numerical stability – it seems that internally some truncation occurs with floating point math. Whilst the results for vanilla Python and Python+Psyco were identical, the results with Shedskin were similar but with fractional divergences in each result.
Whilst these divergences caused some very different results in the final weights for the ANN, my researcher confirms that all the results look equivalent.
Mark Dufour (Shedskin’s author) confirms that Python’s C double is used the same in Shedskin but notes that rounding (or a bug) may be the culprit. Shedskin is a young project, Mark will welcome extra eyes if you want to look into this.
Running the code with Shedskin was fairly easy. On Ubuntu I had to install libgc-dev and libpcre3-dev (detailed in the Shedskin docs) and g++, afterwards shedskin was ready. From download to first run was 15 minutes.
On my first attempt to compile bpnn.py with Shedskin I received an error as the ‘raise’ keyword isn’t yet supported. I replaced the ‘raise’ calls with ‘assert False’ for sanity, afterwards compilation was fine.
Edit – Mark notes that the basic form of ‘raise’ is supported but the version used in bpnn.py isn’t yet supported. Something like ‘raise ValueError(‘some msg’)’ works fine.
Mark notes that Shedskin currently works well up to 500 lines (maybe up to 1000), since bpnn.py is only 200 lines compilation is quick.
Note that if you can’t use Psyco because you aren’t on x86, Shedskin might be useful to you since it’ll work anywhere that Python and g++ compile.
Running this yourself
If you want to recreate my results, download bpnn_shedskin_src_20081117.zip. You’ll see bpnn_shedskin.py, this is the main code. bpnn_shedskin.py includes either ‘examples_short.py’ or ‘examples_full.py’, short is the easier 2 pattern problem and full has 508 patterns.
Note that these patterns are stored as lists of tuples (Shedskin doesn’t support the csv module so I hardcoded the input patterns to speed development), the full version is over 500 lines of Python and this slows Shedskin’s compilation somewhat.
By default the imports for Psyco are commented out and the short problem is configured. At the command line you’ll get an output like this:
python bpnn_shedskin.py Using 2 examples ANN uses 57 input, 50 hidden, 62 output, 1000 iterations, 0.100000 learning rate, 0.000000 momentum error 65.454309 2008-11-17 15:22:58.318593 error 45.176110 2008-11-17 15:22:59.060787 error 44.616933 2008-11-17 15:23:00.246280 error 44.026883 2008-11-17 15:23:01.743821 error 44.049276 2008-11-17 15:23:02.815876 error 44.905183 2008-11-17 15:23:03.860352 error 44.674506 2008-11-17 15:23:05.270307 error 43.365627 2008-11-17 15:23:06.757126 error 43.299160 2008-11-17 15:23:08.244466 error 42.540076 2008-11-17 15:23:09.732035 Elapsed: 0:00:41.472192
If you uncomment the two Psyco lines your code will run about 2.6* faster.
Using Shedskin
To use shedskin, first run the Python through shedskin and then ‘make’ the result. The compiled binary will run much faster than the vanilla Python code, the result below shows the short problem taking 0.19 seconds compared to 41 seconds above.
shedskin bpnn_shedskin.py *** SHED SKIN Python-to-C++ Compiler 0.0.29 *** Copyright 2005-2008 Mark Dufour; License GNU GPL version 3 (See LICENSE) [iterative type analysis..] *** iterations: 3 templates: 519 [generating c++ code..] *WARNING* bpnn_shedskin.py:178: function (class NN, 'weights') not called! *WARNING* bpnn_shedskin.py:156: function (class NN, 'test') not called! make g++ -O2 -pipe -Wno-deprecated -I. -I/usr/lib/shedskin/lib /usr/lib/shedskin/lib/string.cpp /usr/lib/shedskin/lib/random.cpp /usr/lib/shedskin/lib/datetime.cpp examples_short.cpp bpnn_shedskin.cpp /usr/lib/shedskin/lib/builtin.cpp /usr/lib/shedskin/lib/time.cpp /usr/lib/shedskin/lib/math.cpp -lgc -o bpnn_shedskin ./bpnn_shedskin Using 2 examples ANN uses 57 input, 50 hidden, 62 output, 1000 iterations, 0.100000 learning rate, 0.000000 momentum error 65.454309 2008-11-17 16:11:08.452087 error 44.970416 2008-11-17 16:11:08.476869 error 46.444249 2008-11-17 16:11:08.506324 error 44.209054 2008-11-17 16:11:08.519375 error 44.058518 2008-11-17 16:11:08.532430 error 45.655892 2008-11-17 16:11:08.545741 error 44.518816 2008-11-17 16:11:08.558520 error 43.643572 2008-11-17 16:11:08.571705 error 44.800429 2008-11-17 16:11:08.584241 error 43.710905 2008-11-17 16:11:08.597465 Elapsed: 0:00:00.198747
Why is the math different?
An open question remains as to why the evolution of the floating point arithmetic is different between Python and Shedskin. If anyone is interested in delving in to this, I’d be very interested in hearing from you.
Extension modules
Mark notes that the extension module support is perhaps a more useful way to use Shedskin for this sort of problem.
A single module can be compiled (e.g. ‘shedskin -e module.py’) and with Python you just import it (e.g. ‘import module’) and use it…with a big speed-up.
This ties the code to your installed libs – not so great for easy distribution but great for lone researchers needing a speed boost.
Shedskin 0.1 in the works
Mark’s plan is to get 0.1 released over the coming months. One aim is to get the extension module to a similar level of functionality as SWIG and improve the core library support so that Shedskin comes with (some more) Batteries Included.
Mark is open to receiving code (up to 1000 lines) that doesn’t compile. The project would always happily accept new contributors.
See the Shedskin homepage, blog and group.
Ian is a Chief Interim Data Scientist via his Mor Consulting. Sign-up for Data Science tutorials in London and to hear about his data science thoughts and jobs. He lives in London, is walked by his high energy Springer Spaniel and is a consumer of fine coffees.
CNET shows my BrandWatch ProCast
I’m rather proud to say that CNET covered my client BrandWatch and that my 3 minute screencast which introduces BrandWatch’s features is embedded in the article.
The article’s author, Josh, saw my ProCast whilst searching Vimeo for ‘screencasts’. BrandWatch received a great set of referrals from the article and Giles (CEO) is very pleased with the response. I glow.
If you need professional screencasting services, get in contact.
Brighton Python Meet, Weds Oct 29th
We’re having our second Brighton Python (Upcoming) meetup next week on Weds Oct 29th at The Hampton Arms (gmap), a 15 min walk from Brighton station. The pub is 5 minutes from the Churchill shopping Centre and the clocktower off of Western Road (the main shopping road that runs parallel to the sea front).
This is the same location that we used for the last meet and again we’re combining this with the Brighton freelancer Farm meetup for a larger crowd.
Some more details are in this previous post, hope to see you there!
Brighton Python Meetup (Oct 29th)
John and I are organising another Brighton Python meet for the evening of Wednesday Oct 29th (Upcoming for details). [photo by southtyrolean]
We threw one months back along with Paul Silver’s The Farm freelancers, we filled the The Hampton Arms with close to 60 geeks IIRC. The pub is a 15 minute walk from Brighton station (note to GnuBlade and other Londoners, this pub is much closer to the station than the building we use for £5 App meets!).
Amongst others we had Jim of SecondLife UK, a Microsoftie who likes lightweight languages, several pyQT programmers from a medical company and a whole bunch of others.
As before it’ll just be a meet n’greet pub outing, I’m expecting 10-20 Pythonistas along with 20-40 Farmers (they’re PHP, Web, db, graphics etc local freelancers), lots of beer and conversation.
Please sign-up (Upcoming) so we’ve an idea of who will be along.
New ProCast for local BrandWatch
Last week I completed a new ProCasts screencast for local Brand Reputation Management firm BrandWatch, the video is linked on the right of their frontpage (and on my examples page).
The screencast runs for 2 minutes and demos the main features of their app including Trends, Comparisons and how to drill-down into the source stories. The goal is to get more visitors to sign-up to a trial account.
BrandWatch Introduction from IanProCastsCoUk on Vimeo.
I used Barclays and HSBC as my examples, given all the news recently there was a lot of information to work from and BrandWatch neatly pulls out the pertinent articles. I’ve also experimented with more backing music and a call-to-action at the video’s close.
If you need professional screencasting services, get in contact.
Read my book
Oreilly High Performance Python by Micha Gorelick & Ian Ozsvald AI Consulting
Co-organiser
Trending Now
1Leadership discussion session at PyDataLondon 2024Data science, pydata, RebelAI2What I’ve been up to since 2022pydata, Python3Upcoming discussion calls for Team Structure and Buidling a Backlog for data science leadsData science, pydata, Python4My first commit to PandasPython5Skinny Pandas Riding on a Rocket at PyDataGlobal 2020Data science, pydata, PythonTags
Aim Api Artificial Intelligence Blog Brighton Conferences Cookbook Demo Ebook Email Emily Face Detection Few Days Google High Performance Iphone Kyran Laptop Linux London Lt Map Natural Language Processing Nbsp Nltk Numpy Optical Character Recognition Pycon Python Python Mailing Python Tutorial Robots Running Santiago Seb Skiff Slides Startups Tweet Tweets Twitter Ubuntu Ups Vimeo Wikipedia