Entrepreneurial Geekiness
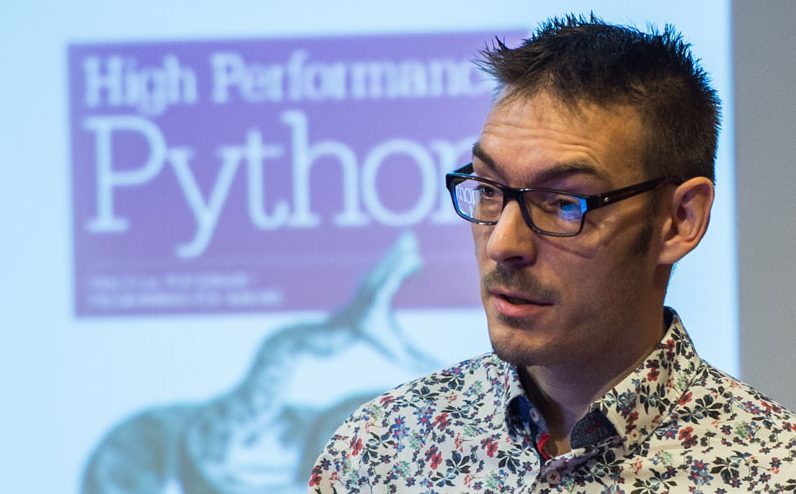
Active Countermeasures for Privacy in a Social Networking age?
This is a bit of a rambling post covering some thoughts on data privacy, mobile phones and social networking.
A general and continued decrease in personal privacy seems inevitable in our age of data (NSA Files at The Guardian). We generate a lot of data, we rarely know how or where it is stored and we don’t understand how easy it is to make certain inferences based on aggregated forms of our data. Cory Doctorow has some points on why we should care about this topic.
Will we now see the introduction of active countermeasures in a data stream by way of protest or camouflage by regular folk?
Update – hat tip to Kyran for prism-break.org, listing open-source alternatives to Operating Systems and communication clients/systems. I had a play earlier today with the Tor-powered Orweb on Android – it Just Worked and whatsmyip.org didn’t know where my device was coming from (running traceroute went from whatsmyip to the Tor entry node and [of course] no further). It seems that installing Tor on a raspberrypi or Tor on EC2 is pretty easy too (Tor runs faster when more people start Tor relays [which carry the internal encrypted traffic, so there’s none of the fear of running an edge nodes that sends the traffic onto the unencrypted Internet]). Here are some Tor network statistic graphs.
I’ve long been unhappy with the fact that my email is known to be transmitted and stored in the clear (accepting that I turn on HTTPS-only in Gmail). I’d really like for it to be readable only for the recipient, not for anyone (sysadmin or Government agency) along the chain. Maybe someone can tell me if adding PGP into Gmail via the browser and Android phone is an easy thing to do?
I’m curious to see how long it’ll be before we have a cypherpunk mobile OS, preconfigured with sensible defaults. CyanogenMod is an open build of Android (so you could double-check for Government backdoors [if you took the time]), there’s no good reason why a distro couldn’t be setup that uses Tor, HTTPSEverywhere (eff.org post on this combo, this Tor blog post comments on Tor vs PRISM) and Incognito Mode by default as a start for private web usage. Add on a secure and open source VoIP client (not Skype) and an IM tool and you’re most of the way there for better-than-normal-folk privacy.
Compared to an iOS device it’ll be a bit clunky (so maybe my mum won’t use it) but I’d like the option, even if I have to jump through a few hoops. You might also choose not to trust your handset provider, we’re just starting to see designs for build-it-yourself cellphones (albeit very basic non-data phones at present).
Maybe we’ll start to consider the dangers of entrusting our data to near-monopolies in the hope that they do no evil (and aren’t subject to US Government secret & uninvestigable
disclosures to people who we personally may or may not trust, and may or may not be decent, upright, solid, incorruptible citizens). Perhaps far-sighted governments in other countries will start to educate their citizens about the dangers of trusting US Data BigCorps (“Loose Lips Sink Ships“)?
So what about active countermeasures? For the social networking example above we’d look at communications traffic (‘friends’ are cheap to acquire but communication takes effort). What if we started to lie about who we talk to? What if my email client builds a commonly-communicated-with list and picks someone from outside of that list, then starts to send them reasonably sensible-looking emails automatically? Perhaps it contains a pre-agreed codeword, then their client responds at a sensible frequency with more made-up but intelligible text. Suddenly they appear to be someone I closely communicate with, but that’s a lie.
My email client knows this so I’m not bothered by it but an eavesdropper has to process this text. It might not pass human inspection but it ought to tie up more resources, forcing more humans to get involved, driving up the cost and slowing down response times. Maybe our email clients then seed these emails with provocative keywords in innocuous phrases (“I’m going to get the bomb now! The bomb is of course the name for my football”) which tie up simple keyword scanners.
The above will be a little like the war on fake website signups for spam being defeated by CAPTCHAs (and in turn defeating the CAPTCHAs), driving perhaps improvements in NLP technologies. I seem to recall that Hari Seldon in Asimov’s Foundation novels used auto-generated plausible speech generators to mask private in-person communications from external eavesdropping (I can’t find a reference – am I making this up?), this stuff doesn’t feel like science fiction any more.
Maybe with FourSquare people will practice fake check-ins. Maybe during a protest you comfortably sit at home and take part in remote virtual check-ins to spots that’ll upset the police (“quick! join the mass check-in in the underground coffee shop! the police will have to spend resources visiting it to see if we’re actually there!”). Maybe you’ll physically be in the protest but will send spoofed GPS co-ords with your check-ins pretending to be elsewhere.
Maybe people start to record and replay another person’s check-ins, a form of ‘identify theft’ where they copy the behaviour of another to mask their own movements?
Maybe we can extend this idea to photo sharing. Some level of face detection and recognition already exists and it is pretty good, especially if you bound the face recognition problem to a known social group. What if we use a graphical smart-paste to blend a person-of-interest’s face into some of our group photos? Maybe Julian Assange appears in background shots around London or a member of Barack Obama’s Government in photos from Iranian photobloggers?
The photos could be small and perhaps reasonably well disguised so they’re not obvious to humans, but obvious enough to good face detection & recognition algorithms. Again this ties up resources (and computer vision algorithms are terribly CPU-expensive). It would no doubt upset the intelligence services if it impacted their automated analysis, maybe this becomes a form of citizen protest?
Hidden Mickeys appear in lots of places (did you spot the one in Tron?), yet we don’t notice them. I’m pretty sure a smart paste could hide a small or distorted or rotated or blended image of a face in some photos, without too much degradation.
Figuring out who is doing what given the absence of information is another interesting area. With SocialTies (built by Emily and I) I could track who was at a conference via their Lanyrd sign-up, and also track people via nearby FourSquare check-ins and geo-tagged tweets (there are plenty of geo-tagged tweets in London…). Inferring where you were was quite possible, even if you only tweeted (and had geo-locations enabled). Double checking your social group and seeing that friends are marked as attending the event that you are near only strengthens the assertion that you’re also present.
Facebook typically knows the address book of your friends, so even if you haven’t joined the service it’ll still have your email. If 5 members of Facebook have your email address then that’s 5 directed edges in a social network graph pointing at a not-yet-active profile with your name on it. You might never join Facebook but they still have your email, name and some of your social connections. You can’t make those edges disappear. You just leaked your social connectivity without ever going near the service.
Anyhow, enough with the prognostications. Privacy is dead. C’est la vie. As long as we trust the good guys to only be good, nothing bad can happen.
Ian is a Chief Interim Data Scientist via his Mor Consulting. Sign-up for Data Science tutorials in London and to hear about his data science thoughts and jobs. He lives in London, is walked by his high energy Springer Spaniel and is a consumer of fine coffees.
Open Sourcing “The Screencasting Handbook”
Back in 2010 I released the finished version of my first commercial eBook The Screencasting Handbook. It was 129 pages of distilled knowledge for the budding screencaster, written in part to introduce my (then) screencasting company ProCasts to the world (which I sold years back) and based on experience teaching through ShowMeDo. Today I release the Handbook under a Creative Commons License. After 3 years the content is showing its age (the procedures are good, the software-specific information is well out of date), I moved out of screencasting a while back and have no plans to update this book.
The download link for the open sourced version is at thescreencastinghandbook.com.
I’m using the Creative Commons Unported license – it allows anyone to derive a new version and/or make commercial usage without requiring any additional permissions from me, it does require attribution. This is the most open license I can give that still gives me a little bit of value (by way of attribution). The license must not be modified.
If someone would like to derive an updated version (with or without a price tag) you are very welcome to – just remember to attribute back to the original site and to this site with my name please (as noted at the download point). You can not change the license (but if you wanted to make a derived and non-open-source version of the book for commercial use, I’m sure we can come to an arrangement).
Previously I’ve discussed how I wrote the Handbook in an open, collaborative fashion (with monthly chapter releases to the preview audience), this was a good procedure that I’d use again. Other posts discussing the Handbook are under the “screencasting-handbook” tag.
Ian is a Chief Interim Data Scientist via his Mor Consulting. Sign-up for Data Science tutorials in London and to hear about his data science thoughts and jobs. He lives in London, is walked by his high energy Springer Spaniel and is a consumer of fine coffees.
Social Media Brand Disambiguator first steps
As noted a few days back I’m spending June working on a social-media focused brand disambiguator using Python, NLTK and scikit-learn. This project has grown out of frustrations using existing Named Entity Recognition tools (like OpenCalais and DBPediaSpotlight) to recognise brands in social media messages. These tools are generally trained to work on long-form clean text and tweets are anything but long or cleanly written!
The problem is this: in a short tweet (e.g. “Loving my apple, like how it werks with the iphon”) we have little context to differentiate the sense of the word “apple”. As a human we see the typos and deliberate spelling errors and know that this use of “apple” is for the brand, not for the fruit. Existing APIs don’t make this distinction, typically they want a lot more text with fewer text errors. I’m hypothesising that with a supervised learning system (using scikit-learn and NLTK) and hand tagged data I can outperform the existing APIs.
I started on Saturday (freshly back from honeymoon), a very small github repo is online. Currently I can ingest tweets from a JSON file (captured using curl), marking the ones with a brand and those with the same word but not-a-brand (in-class and out-of-class) in a SQLite db. I’ll benchmark my results against my hand-tagged Gold Standard to see how I do.
Currently I’m using my Python template to allow environment-variable controlled configurations, simple logging, argparse and unittests. I’ll also be using the twitter text python module that I’m now supporting to parse some structure out of the tweets.
I’ll be presenting my progress next week at Brighton Python, my goal is to have a useful MIT-licensed tool that is pre-trained with some obvious brands (e.g. Apple, Orange, Valve, Seat) and software names (e.g. Python, vine, Elite) by the end of this month, with instructions so anyone can train their own models. Assuming all goes well I can then plumb it into my planned annotate.io online service later.
Ian is a Chief Interim Data Scientist via his Mor Consulting. Sign-up for Data Science tutorials in London and to hear about his data science thoughts and jobs. He lives in London, is walked by his high energy Springer Spaniel and is a consumer of fine coffees.
Thoughts from a month’s backpacking honeymoon
I’m publishing this on the hoof, right now we’re in Istanbul near the end of our honeymoon back home. Here are some app-travelling notes (for our Nexus 4 Androids).
Google Translate offers Offline dictionaries for all the European languages, each is 150mb. We downloaded new ones before each country hop. Generally they were very useful, some phrases were wrong or not colloquial (often for things like “the bill please”). Some languages had pronunciation guides, they were ok but a phrase book would be better. It worked well as a glorified language dictionary.
Google Maps Offline were great except Hungary where offline wasn’t allowed (it didn’t explain why).
The lack of phrase or dictionary apps was a pain, there’s a real dearth on Android. Someone should fill this gap!
WiFi was fairly common throughout our travels so we rarely used our paper Guides. WiFi was free in all hotels, sometimes in train stations, often in cafes and bars even in Romania.
WikiSherpa caches recent search results which are pulled out of Wikipedia and Wikivoyage, this works like a poor man’s RoughGuide. It doesn’t link to any maps or cache images but if you search on a city, you can read up on it (e.g. landmarks, how to get a taxi etc) whilst you travel.
The official WikiPedia app has page saving, this is useful for background info on a city when reading offline.
AnyMemo is useful for learning phrases in new languages. It is chaotic as the learning files aren’t curated. You can edit the files to remove the phrases you don’t need and to add useful new ones in.
Emily notes that TripAdvisor on Android doesn’t work well (the iPhone version was better but still not great). Emily also notes that hotels.com, lastminute and booking.com were all useful for booking most of our travels and hotels.
We used foursquare when we had WiFi, sadly there is no offline mode so I just starred locations using Google Maps. Foursquare needs a language independent reading system, trying to figure out if a series of Turkish reviews were positive or not based on the prevalence of smileys wasn’t easy (Google Translate integration would have helped). An offline FourSquare would have been useful (e.g. for cafes near to our spot).
We really should have bought a WiFi 3G dongle. The lack of data was a pain. We used Emily’s £5 travel data day plans on occasion (via Three). It works for most of Europe but not Switzerland or Turkey.
Given that we have WikiPedia and Wiktionary, how come we don’t have a “WikiPhrases” (“wikilingo”?) with multi-language forms of common phrases? Just like the phrase books for travel that we can buy but with good local phrases and idioms across any language that gets written up. This feels like it’d have a lot of value.
Ian is a Chief Interim Data Scientist via his Mor Consulting. Sign-up for Data Science tutorials in London and to hear about his data science thoughts and jobs. He lives in London, is walked by his high energy Springer Spaniel and is a consumer of fine coffees.
June project: Disambiguating “brands” in Social Media
Having returned from Chile last year, settled in to consulting in London, got married and now on honeymoon I’m planning on a change for June.
I’m taking the month off from clients to work on my own project, an open sourced brand disambiguator for social media. As an example this will detect that the following tweet mentions Apple-the-brand:
“I love my apple, though leopard can be a pain”
and that this tweet does not:
“Really enjoying this apple, very tasty”
I’ve used AlchemyAPI, OpenCalais, DBPedia Spotlight and others for client projects and it turns out that these APIs expect long-form text (e.g. Reuters articles) written with good English.
Tweets are short-form, messy, use colloquialisms, can be compressed (e.g. using contractions) and rely on local context (both local in time and social group). Linguistically a lot is expressed in 140 characters and it doesn’t look like”good English”.
A second problem with existing APIs is that they cannot be trained and often don’t know about European brands, products, people and places. I plan to build a classifier that learns whatever you need to classify.
Examples for disambiguation will include Apple vs apple (brand vs e.g. fruit/drink/pie), Seat vs seat (brand vs furniture), cold vs cold (illness vs temperature), ba (when used as an abbreviation for British Airways).
The goal of the June project will be to out-perform existing Named Entity Recognition APIs for well-specified brands on Tweets, developed openly with a liberal licence. The aim will be to solve new client problems that can’t be solved with existing APIs.
I’ll be using Python, NLTK, scikit-learn and Tweet data. I’m speaking on progress at BrightonPy and DataScienceLondon in June.
Probably for now I should focus on having no computer on my honeymoon…
Ian is a Chief Interim Data Scientist via his Mor Consulting. Sign-up for Data Science tutorials in London and to hear about his data science thoughts and jobs. He lives in London, is walked by his high energy Springer Spaniel and is a consumer of fine coffees.
Read my book
Oreilly High Performance Python by Micha Gorelick & Ian Ozsvald AI Consulting
Co-organiser
Trending Now
1Leadership discussion session at PyDataLondon 2024Data science, pydata, RebelAI2What I’ve been up to since 2022pydata, Python3Upcoming discussion calls for Team Structure and Buidling a Backlog for data science leadsData science, pydata, Python4My first commit to PandasPython5Skinny Pandas Riding on a Rocket at PyDataGlobal 2020Data science, pydata, PythonTags
Aim Api Artificial Intelligence Blog Brighton Conferences Cookbook Demo Ebook Email Emily Face Detection Few Days Google High Performance Iphone Kyran Laptop Linux London Lt Map Natural Language Processing Nbsp Nltk Numpy Optical Character Recognition Pycon Python Python Mailing Python Tutorial Robots Running Santiago Seb Skiff Slides Startups Tweet Tweets Twitter Ubuntu Ups Vimeo Wikipedia