Entrepreneurial Geekiness
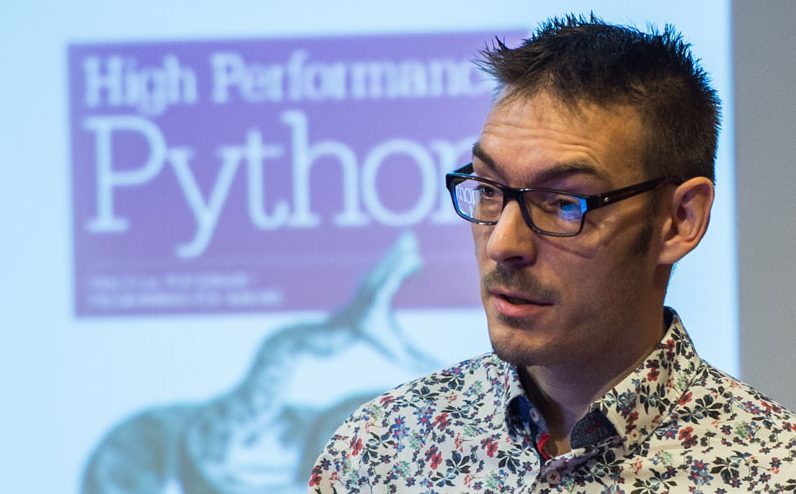
Annotate.io self-learning text cleaner demo online
A few weeks I posted some notes on a self-learning text cleaning system, to be used by data scientists who didn’t want to invest time cleaning their data by hand. I have a first demo online over at annotate.io (the demo code is here in github).
The intuition behind this is that we currently divert a lot of mental resource early in a project to cleaning data and a bunch of that can be spent just figuring out which libraries will help with the cleaning. What if we could just let the machine do that for us? We can then focus on digging into new data and figuring out how to solve the bigger problems.
With annotate.io you give it a list of “data you have” and “data you want”, it’ll figuring out how to transform the former into the latter. With the recipe it generates you then feed in new data and it performs the cleaning for you. You don’t have to install any of the libraries it might use (that’s all server-side).
Using Python 2.7 or 3.4 you can run the demo in github (you need the requests library). You can sign-up to the announce list if you’d like to be kept informed on developments.
Ian is a Chief Interim Data Scientist via his Mor Consulting. Sign-up for Data Science tutorials in London and to hear about his data science thoughts and jobs. He lives in London, is walked by his high energy Springer Spaniel and is a consumer of fine coffees.
Data Science Jobs UK (ModelInsight) – Python Jobs Email List
I’ve had people asking me about how they can find data scientists in London and through our PyDataLondon meetup we’ve had members announcing jobs. There’s no central location for data science jobs so I’ve put together a new list (administered through my ModelInsight agency).
Sign-up to the list here: Data Science Jobs UK (ModelInsight)
- Aimed at Data Science jobs in the UK
- Mostly Python (maybe R, Matlab, Julia if relevant)
- It’ll include Permie and Contract jobs
The list will only work if you can trust it so:
- Your email is private (it is never shared)
- The list is on MailChimp so you can unsubscribe at any time
- We vet the job posts and only forward them if they’re in the interests of the list
- Nobody else can post into the list (all jobs are forwarded just by us)
- It’ll be low volume and all posts will be very relevant
Sign-up to the list here: Data Science Jobs UK (ModelInsight)
Obviously if you’re interested in joining the London Python data science community then come along to our PyDataLondon meetups.
Ian is a Chief Interim Data Scientist via his Mor Consulting. Sign-up for Data Science tutorials in London and to hear about his data science thoughts and jobs. He lives in London, is walked by his high energy Springer Spaniel and is a consumer of fine coffees.
A first approach to automatic text data cleaning
In October I gave the opening keynote at PyConIreland on The Real Unsolved Problems in Data Science. One of the topics I covered was poor quality data, by some estimates data cleaning occupies 50-80% of a data scientist’s time.
Personally I’ve just spent the better part of last year figuring out ways to convert poorly-represented company names on 100,000s CVs/resumes to a cleaned subset for my contract recruitment client (via my ModelInsight). This enables us to build ranking engines for contract job applicants (and I’ll note happily that it works rather well!). It only works because we put so much effort into cleaning the raw data. Huge investments like this are expensive in time and money, that carries risk for a client. Tools used include NLTK, ftfy, Pandas, scikit-learn and the re module, all in Python 3.4.
During the keynote I asked if anyone had tooling they could open up to make this sort of task easier. I didn’t get a lot of feedback on that so I’ve had a crack at one of the problems I’d discussed on my annotate.io.
The mapping of raw input data to a lower-dimensional output isn’t trivial, but it felt like something that might be automated. Let’s say you scraped job adverts (e.g. using import.io on adzuna, both based in London). The salary field for the jobs will be messy, it’ll include strings like “To 53K w/benefits”, “30000 OTE plus bonus” and maybe even non-numeric descriptions like “Forty two thousand GBP”. Theses strings are collated from a diverse set of job adverts, all typed by hand by a human and there’s no standard format.
Let’s say we’re after “53000”, “30000”, “42000” as an output. We can expand contractions (“<nbr>K”->”<nbr>000), convert written numbers into an integer and then extract the number. If you’re used to this sort of process then you might expect to spend 30-60 minutes writing unit tests and support code. When you come to the next challenge, you’ll repeat that hour or so of work. If you’re not sure how you want your output data to look you might spend considerably longer trying transformation ideas. What if we could short-circuit this development process and just focus on “what we have” and “what we want”?
More complex tasks include transforming messy company name strings, fixing broken unicode and converting unicode to ASCII (which can ease indexing for search) and identifying tokens that need to be stripped or transformed. There’s a second example over at Annotate and more will follow. I’m about to start work on ‘fact extraction’ – given a block of text (e.g. a description field) can we reliably extract a single fact that’s written in a variety of ways?
Over at Annotate.io I’ll be uploading the first version of a learning text transformer soon. It takes a set of example input->output mappings, learns a transformation sequence that minimizes the transformation distance (hopefully to a distance of 0 meaning it has solved the problem) and then it can use this transformation sequence on future text you pass into the system.
The API is JSON based and will come with Python examples, there’s a mailing list you can join on the site for announcements. I’m specifically interested in the kind of problems you might want to put into this system, please get in contact if you’re curious.
I’m also hoping to work on another data cleaning tool later. If you want to talk about this at a future PyDataLondon meetup, I’d love to chat.
Ian is a Chief Interim Data Scientist via his Mor Consulting. Sign-up for Data Science tutorials in London and to hear about his data science thoughts and jobs. He lives in London, is walked by his high energy Springer Spaniel and is a consumer of fine coffees.
New Relic, uWSGI and “Cannot perform a data harvest for ‘‘ as there is no active session.”
This is more a note-to-self and maybe to another confused soul – if you’re using New Relic (it seems to be really rather nice for web app monitoring) with uWSGI, by default uWSGI runs without the GIL. This means no threads and this means New Relic won’t report anything which leads to a confusing first try.
Specifically read the Best Practices notes for uWSGI around “–enable-threads”. You have to add “–enable-threads” if you’re using New Relic’s Python agent, this is documented on their Python Agent Integration docs for uWSGI but for me the clue was in their log (by default in /tmp/newrelic-python-agent.log if you enable it in newrelic.ini) which showed:
(3717/NR-Harvest-Thread) newrelic.core.agent DEBUG - Commencing harvest of all application data. (3717/NR-Harvest-Thread) newrelic.core.application DEBUG - Cannot perform a data harvest for '<appname>' as there is no active session. (3717/NR-Harvest-Thread) newrelic.core.agent DEBUG - Completed harvest of all application data in 0.00 seconds.
Once I’d added “–enable-threads” to uWSGI the logs looked a lot healthier, particularly:
(3292/NR-Harvest-Thread) newrelic.core.agent DEBUG - Commencing harvest of all application data. (3292/NR-Harvest-Thread) newrelic.core.application DEBUG - Commencing data harvest of '<appname>'. ...
(3292/NR-Harvest-Thread) newrelic.core.application DEBUG - Send profiling data for harvest of '<appname>'. (3292/NR-Harvest-Thread) newrelic.core.application DEBUG - Done sending data for harvest of '<appname>'.
Ian is a Chief Interim Data Scientist via his Mor Consulting. Sign-up for Data Science tutorials in London and to hear about his data science thoughts and jobs. He lives in London, is walked by his high energy Springer Spaniel and is a consumer of fine coffees.
We’re running more Data Science Training in 2015 Q1 in London
A couple of weeks ago Bart and I ran two very successful training courses in London through my ModelInsight, one introduced data science using pandas and numpy to build a recommender engine, the second taught a two-day course on High Performance Python (and yes, that was somewhat based on my book with a lot of hands-on exercises). Based on feedback from those courses we’re looking to introduce up to 5 courses at the start of next year.
If you’d like to hear about our London data science training then sign-up to our (very low volume) announce list. I posted an anonymous survey onto the mailing list, if you’d like to give your vote to the courses we should run then jump over here (no sign-up, there’s only 1 question, there’s no commitment).
If you’d like to talk about these in person then you can find me (probably on-stage) co-running the PyDataLondon meetups.
Here’s the synopses for each of the proposed courses:
“Playing with data – pandas and matplotlib” (1 day)
Aimed at beginner Pythonista data scientists who want to load, manipulate and visualise data
We’ll use pandas with many practical exercises on different sorts of data (including messy data that needs fixing) to manipulate, visualise and join data. You’ll be able to work with your own data sets after this course, we’ll also look at other visualise tools like Seaborn and Bokeh. This will suit people who haven’t used pandas who want a practical introduction such as data journalists, engineers and semi-technical managers.
“Building a recommender system with Python” (1 day)
Aimed at intermediate Pythonistas who want to use pandas and numpy to build a working recommender engine, this covers both using data through to delivering a working data science product. You already know a little linear algebra and you’ve used numpy lightly, you want to see how to deploy a working data science product as a microservice (Flask) that could reliably be put into production.
“Statistics and Big Data using scikit-learn” (2 days)
Aimed at beginner/intermediate Pythonistas with some mathematical background and a desire to learn everyday statistics and to start with machine learning
Day 1 – Probability, distributions, Frequentist and Bayesian approaches, Inference and Regression, Experiment Design – part discussion and part practical
Day 2 – Applying these approaches with scikit-learn to everyday problems, examples may include (note *examples may change* this just gives a flavour) Bayesian spam detection, predicting political campaigns, quality testing, clustering, weather forecasting, tools will include Statsmodels and matplotlib.
“Hands on with Scikit-Learn” (5 days)
Aimed at intermediate Pythonistas who need a practical and comprehensive introduction to machine learning in Python, you’ve already got a basic statistical and linear algebra background
This course will cover all the terminology and stages that make up the machine learning pipeline and the fundamental skills needed to perform machine learning successfully. Aided by many hands on labs with Python scikit-learn the course will enable you to understand the basic concepts, become confident in applying the tools and techniques, and provide a firm foundation from which to dig deeper and explore more advanced methods.
“High Performance Python” (2 days)
Aimed at intermediate Pythonistas whose code is too slow
Day 1 – Profiling (CPU and RAM), compiling with Cython, using Numba, PyPy and Pythran (all the way through to using OpenMP)
Day 2 – Going multicore (multiprocessing) and multi-machine (IPython parallel), fitting more into RAM, probabilitistic counting, storage engines, Test Driven Development and several debugging exercises
A mix of theory and practical exercises, you’ll be able to use the main Python tools to confidently and reliably make your code run faster
Ian is a Chief Interim Data Scientist via his Mor Consulting. Sign-up for Data Science tutorials in London and to hear about his data science thoughts and jobs. He lives in London, is walked by his high energy Springer Spaniel and is a consumer of fine coffees.
Read my book
Oreilly High Performance Python by Micha Gorelick & Ian Ozsvald AI Consulting
Co-organiser
Trending Now
1Leadership discussion session at PyDataLondon 2024Data science, pydata, RebelAI2What I’ve been up to since 2022pydata, Python3Upcoming discussion calls for Team Structure and Buidling a Backlog for data science leadsData science, pydata, Python4My first commit to PandasPython5Skinny Pandas Riding on a Rocket at PyDataGlobal 2020Data science, pydata, PythonTags
Aim Api Artificial Intelligence Blog Brighton Conferences Cookbook Demo Ebook Email Emily Face Detection Few Days Google High Performance Iphone Kyran Laptop Linux London Lt Map Natural Language Processing Nbsp Nltk Numpy Optical Character Recognition Pycon Python Python Mailing Python Tutorial Robots Running Santiago Seb Skiff Slides Startups Tweet Tweets Twitter Ubuntu Ups Vimeo Wikipedia